Dolby researchers Guanxin Jiang and Arijit Biswas were part of the team that co-authored the paper “InSE-NET: A perceptually coded audio quality model based on CNN” that won Best Student Paper for the 151st Audio Engineering Society convention. We gathered a few Dolby researchers together to help answer questions about audio quality, deep learning, and the research methods discussed in that peer-recognized paper.
Understanding audio quality is foundational to delivering high-quality audio in services ranging from real-time streaming, to music mastering, communications, and media post-production,
Understanding Audio Quality
At Dolby, we are inspired by the creativity of artists, filmmakers, musicians, and storytellers. It is our mission to deliver artistic intent to billions of consumers across the world so that they can experience exactly what the artist intended.
Why does audio quality matter?
Audio is a key component to a magical experience. Modern media consumers are becoming even more savvy about audio technology and expect a high-quality listening experience. They are using increasingly high-resolution and high-fidelity systems both on mobile devices and in their living rooms. Therefore, audio quality matters for both Dolby’s mission and for the enjoyment of billions of consumers across the globe.
This passion is behind the wide range of APIs, SDKs, and technologies Dolby provides to both developers who build applications and our partners who develop consumer products.
From images to audio quality
As a student, Guanxin specialized in studying medical image processing and now applies some of that know-how with deep learning techniques to the audio domain.
Fundamentally both images and audio are signals, with the former being a 2D signal and the latter being a 1D signal. Often, audio signals are transformed into spectrograms (see examples below) for signal analysis. With spectrograms presented as an image, the x-axis represents time and the y-axis represents audio frequency. See Visualizing audio with a spectrogram in Python for an example of how to automate making one. Signal processing by deriving hand-crafted features for further analysis is possible while controlling other downstream audio processing and classification tasks.
The strength of deep learning however is to work directly with raw signal samples without using any hand-crafted features. This is challenging for audio for a few reasons.
(1) Audio has a high dimensionality
It is possible to identify the content of an image from a 64×64 image, but the substance of audio may not be recognizable from an equivalent number of samples.
(2) Audio is temporal
With audio, there is a multi-scale temporal dependency on the interpretation of a frequency and the order in which it occurs.
(3) Perception of sound isn’t uniform
Two audio waveforms may look completely different, but may sound exactly the same. The below three samples show two sounds being compared.
Example 1: Two audio clips that generate the same waveform appearance but have different sound impressions and spectrograms.
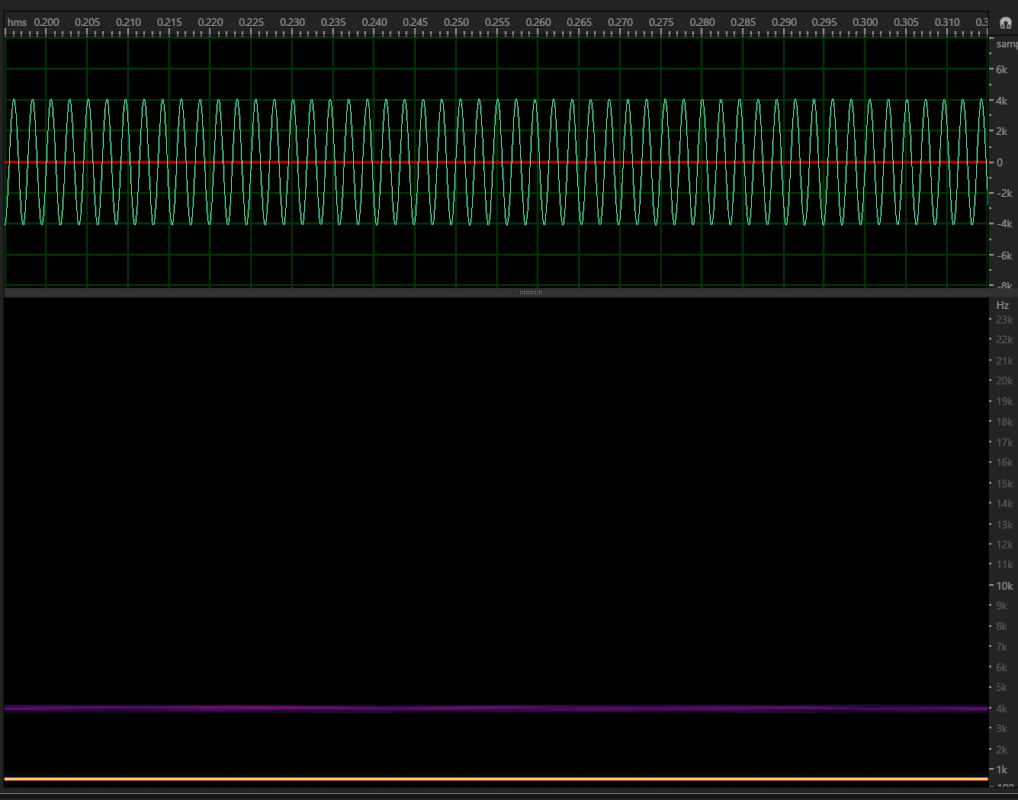
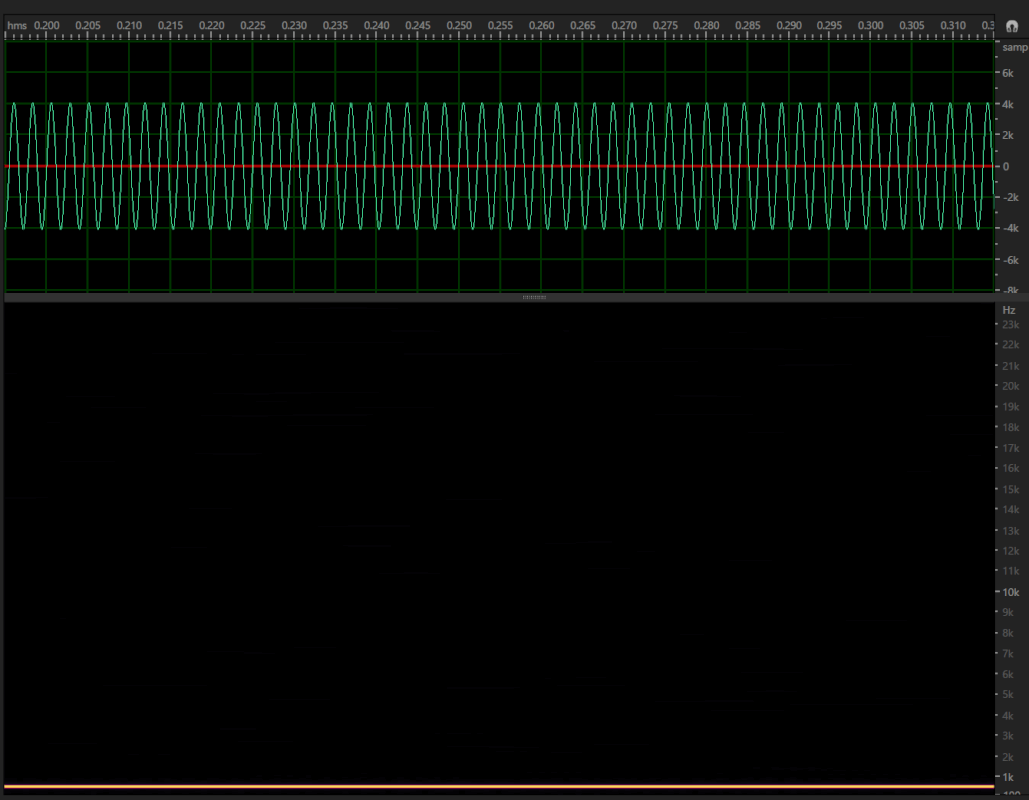
Example 2: Two audio clips that generate the same spectrogram but have different sound impressions and waveforms.
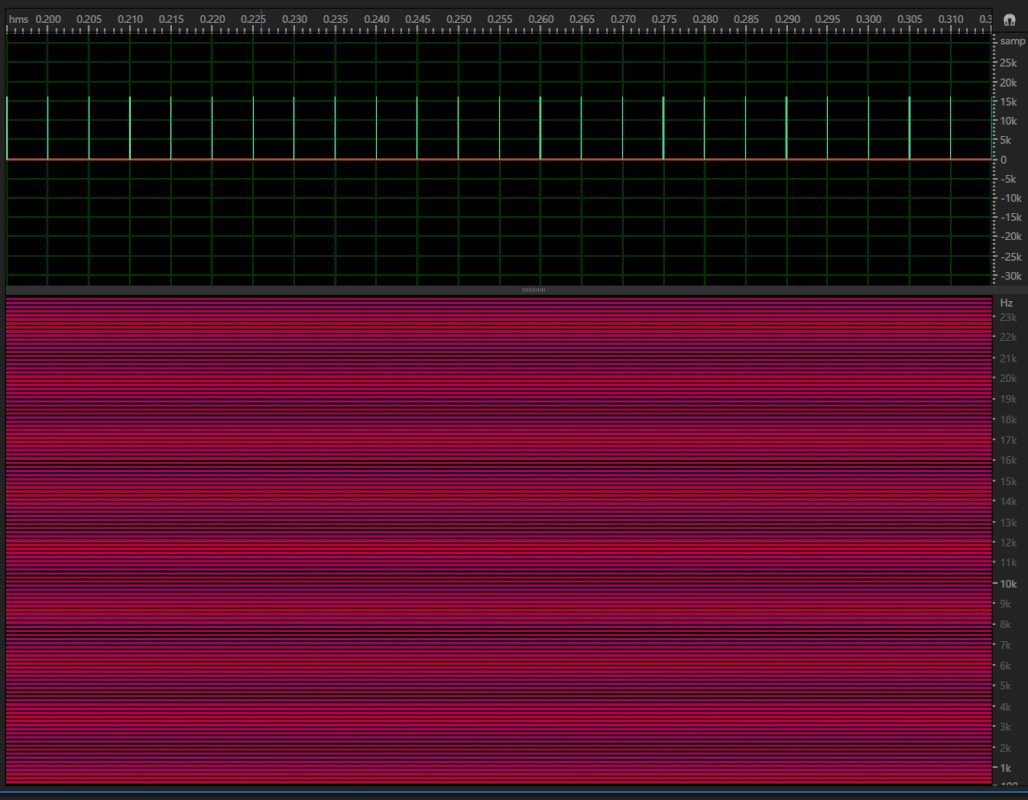
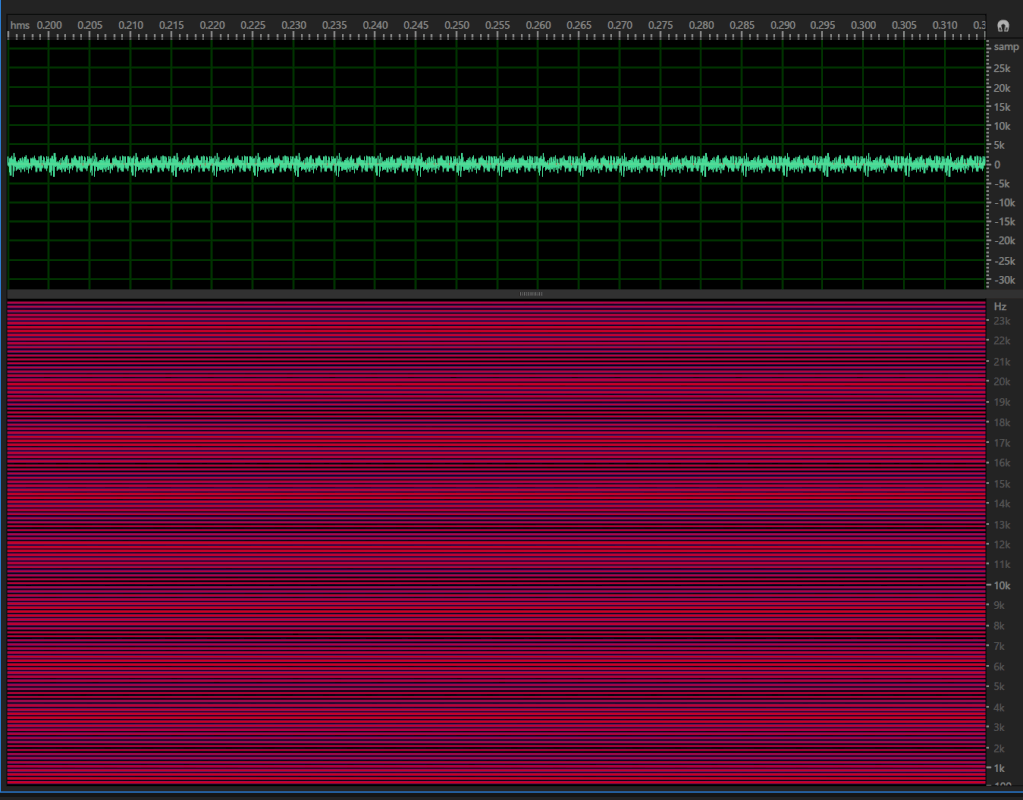
Example 3: Two audio clips that sound the same but generate different spectrograms and waveforms.
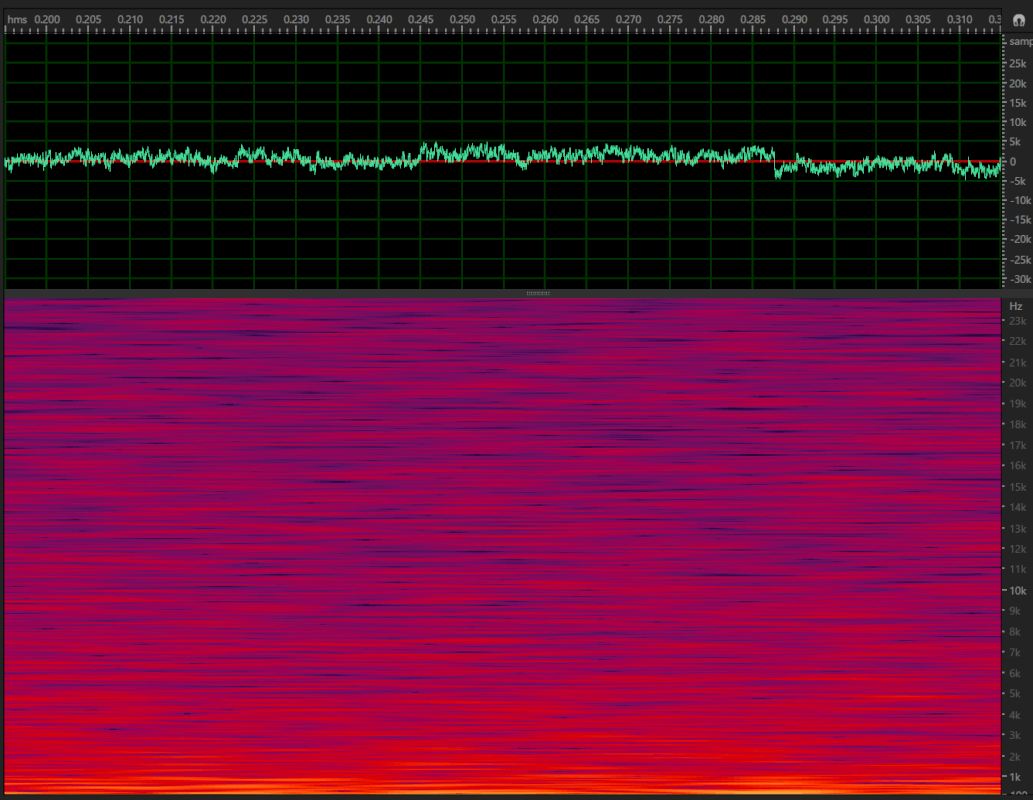
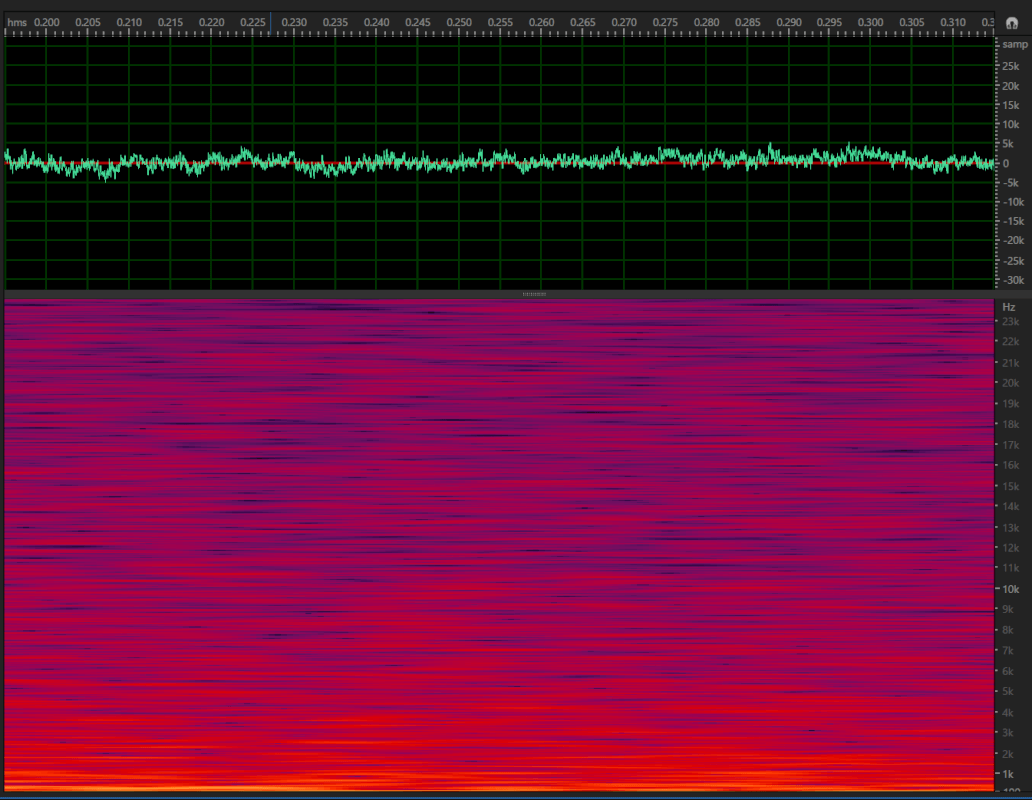
To overcome these challenges, audio is often transformed into an image-like representation and then we can use “image-inspired” networks. In this sense, one can understand relationships in audio by analyzing the visual representation as an image.
Over the past few years several deep networks were designed specifically for audio so it is an area of continued research.
Hardware Impact on Audio Quality
It is difficult to quantity how much software or hardware can impact audio quality. Perception requires an end-to-end system though, changing the microphone or speakers alone may not have the full impact.
Just as we care about shipping audio codecs that deliver artistic intent to consumers, it’s also the responsibility of the device manufacturers (e.g., smart speakers, TVs, etc.) to deliver the best audio experience to their customers. Any audio processing built into their hardware at the end of the chain should not break the promise of delivering the “artistic-intent” to the consumers. Therefore, quite often these device manufacturers work very closely with Dolby for guaranteed reproduction of the artist’s intent.
Subjective Listening Tests
Subjective listening tests are the “golden-standard” for evaluating audio. Sound-quality evaluation tests are critical since they provide the necessary user feedback that drives our research and development. In every step from research to production, we conduct carefully designed subjective listening tests. However, one needs to invest time and expense to obtain the “gold standard” level of human judgments.
Subjective testing methodologies and design strongly depends on the application. For example, when developing a new audio codec from scratch or tuning an existing audio encoder with new settings, it is an important task to evaluate the perceived audio quality. For such subjective tests, we typically use the standardized Multiple Stimuli with Hidden Reference and Anchor (MUSHRA) listening tests. These tests include an unencoded hidden reference, the 3.5 kHz and 7 kHz low-pass filtered versions of the unencoded signals, and one or more coded signals. Subjects are then tasked to rate the signals on a 0-100 MUSHRA quality scale; a well-established quality scale for assessing the quality of audio codecs.
For conducting such tests, apart from sophisticated listening rooms, one needs a user interface for subjects to use.
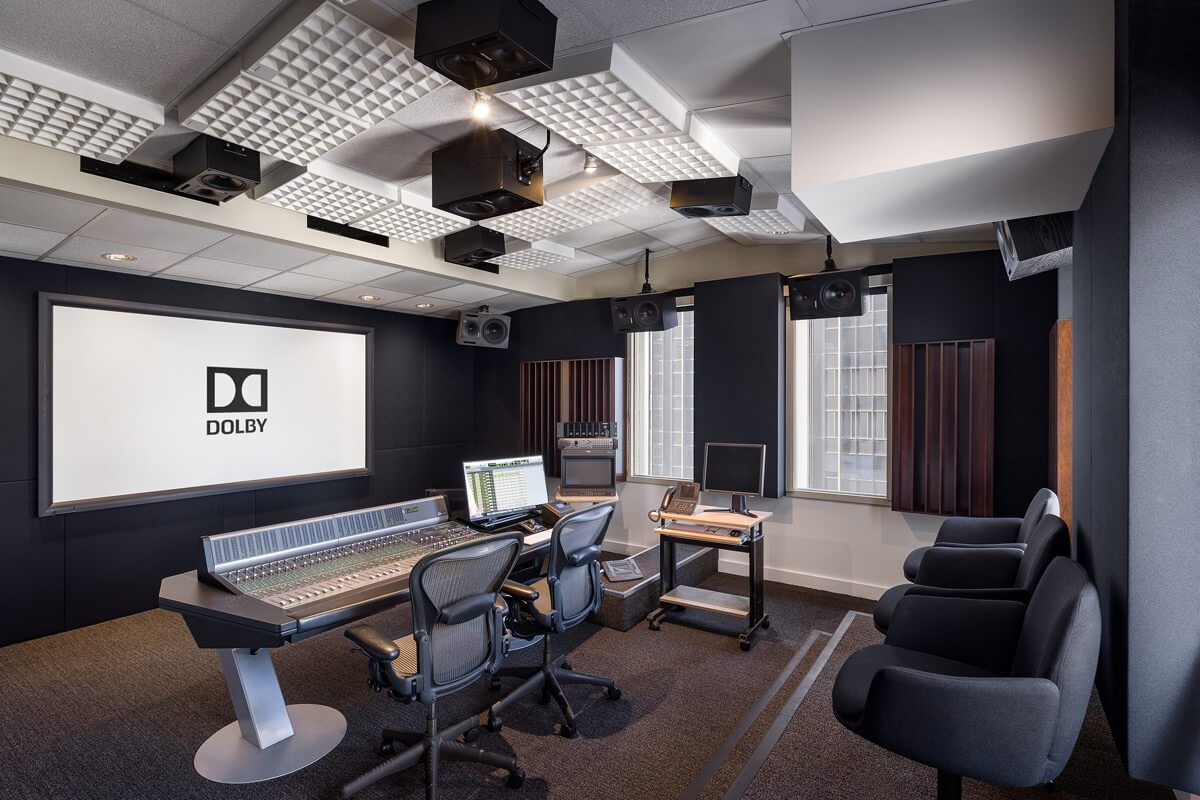
Deep Learning for Better Audio
Signal processing by experts was common practice for many years. The application of Deep Learning techniques common in Natural Language Processing (NLP) and Computer Vision has led to some fascinating use cases.
“Hello World” for Deep Learning
A well-known “Hello world” for deep learning on images is the handwritten digit classification (trained with the MNIST dataset). You gather your model and a collection of hand-written numbers from 0 to 9, and the model can predict the number.
For audio, there is no such well-known task. The audio community has tried to build tasks based on a similar spirit as the handwritten digit recognition task, e.g., spoken digit recognition. Convert spoken digits to some perceptually motivated spectrograms, treat them as an image, and train a classifier to identify the digits.
Audio Data for Analysis
This is the most important aspect for any deep learning-based system. Intuitively, you may be tempted to grab all the subjective listening test data (which a company like Dolby has in abundance) and train a model to predict the subjective quality. However, if you take that approach, you’ll soon realize that it requires a significant manual effort to gather, clean up and organize the data before you could start training your model.
Mind you, when subjective tests are done, the intention was not to develop a quality metric. Anyone who is well versed in machine learning can imagine if the audio content is not diverse enough and does not cover a big range of audio distortions for the range of applications you’re interested in, most likely the model will not be robust in real-world applications. This was the reason, we trusted our expertise in the perceptual frontend, and simply asked the model following it to mimic an existing quality metric with diverse data and distortions. This not only helped us to get started quickly but we started getting competitive results. Then, in our next paper, we trained our model with listening tests, which gave a further boost in prediction accuracy.
Synthetic data may not be analogous to real-world datasets unless it’s engineered with care. Let’s take the example of audio quality label associated with a compressed audio with respect to an uncompressed audio. Subjective test data with diverse audio excerpts are scarce and collecting reliable listening results with quality labels is time-consuming.
To train deep models for predicting the quality, one needs a large training dataset. Hence, it is common for domain experts to engineer synthetic data. Unless someone has sufficient experience in making an educated guess about expected quality based on underlying audio compression algorithms, it’s difficult to create reliable synthetic dataset programmatically.
Quality Features and Artifacts
Different types of audio processing introduce different types of artifacts. For example, audio compression may introduce a certain type of artifacts whereas a speech production chain can itself introduce certain other type artifacts. We can enhance audio to correct for issues, as discussed in Building a Speech Enhancement Chain.
Currently, an area of focus is on predicting the quality after compressing (aka coding) audio, given the reference un-compressed audio. Even here, different types of audio codecs, and audio coding tools (i.e., processing blocks in a given codec) may introduce a certain unique kind of artifact. To learn more about the artifacts introduced by audio compression techniques along with corresponding audio examples, can be found in the comprehensive tutorial created by the AES Technical Committee on Coding of Audio Signals.
A very simplistic example is as follows. If you drastically reduce the bitrate from a very high bitrate to a very low bitrate, the most obvious change that you would notice will be a reduction in bandwidth. The audio will sound muffled; and if the sound was enveloping you, after the drastic bandwidth bitrate reduction, spatially the audio will sound narrow. Like humans, these drastic changes can be easily detected by a machine but monitoring the subtle subjective changes in quality with bitrates is where the challenge is.
As developers of the model, one needs to be “critical listeners” and not trust what the model is predicting, or else it is easy to get biased by the model itself and realize later you missed an important bug (either in the training dataset design or in the code itself).
In short, the main challenge is one needs to have a multi-disciplinary skillset as well as a mindset.
Key Tools and Technologies
Our deep learning training setup is implemented with PyTorch, which you may know is a Pythonic deep learning framework. Thus, much of our code on this project was written in Python and PyTorch.
The model architecture of InSE-Net is quite compact and the computation on GPUs/TPUs is not the issue. Even a reasonably powered GPU (e.g., Nvidia GeForce GTX 1080 Ti) is sufficient to train the model we presented in our two AES papers. Even if we have to deal with less powerful GPUs, DataParallel and DistributedDataParallel functions in PyTorch support splitting the input across several devices with multi-threading.
The real culprit of many deep learning trainings, also in our case, is the data throughput. Data pre-processing such as some data augmentation techniques are done on CPUs. Data loading from CPUs to GPUs are the real bottleneck. To avoid repetitive computation on CPUs for each time re-training a new model, we pre-computed and saved all the spectrograms of reference and distorted audio data of the training dataset. PyTorch also has a Dataloader abstraction that takes care of batching and pre-loading with an extra argument num_workers.
Running the model in test mode is as simple as calling Python script with reference and distorted audio file (or directory) as inputs, and out comes the quality score of the distorted audio (or a batch of distorted audio in a directory). The test mode can run either on a CPU or on a GPU on any modern PC/Laptop.
Continued Perception Research and Applications
At Dolby, we are lucky to have so many talented researchers around the company, who are internationally renowned experts not only in the field of the science of sound and hearing but also in audio compression, deep learning and AI. Inspiration from our scientific expertise inside the company, coupled with our passion for ensuring the delivery of high-quality audio to consumers all over the world, fuels our desire to continue researching how to guarantee a proper listening experience on a variety of devices.
Check out our Audio Research page for more areas of active research. You will also find audio quality is foundational to delivering high-quality interactive, social, and immersive experiences such as applications in real-time streaming, music mastering, communications, and media post-production.
Additional References
To read the original paper that inspired this post, visit the AES e-Library:
- https://www.aes.org/e-lib/browse.cfm?elib=21478 (InSE-NET)
- https://www.aes.org/e-lib/browse.cfm?elib=21902 (Stereo InSE-NET)
Several additional citations referenced in the papers can be worth a read for somebody that has an interest in a deeper dive into the subject.
This paper gives a very high-level description of audio coding with some puzzling results: Learning about perception of temporal fine structure by building audio codecs.